For years, medicine has struggled to treat antibiotic-resistant bacteria, like MRSA, which I wrote about in my February 12th article. However, machine learning could potentially revolutionize—not to mention speed up—the way in which researchers find successful antibiotic candidates. Experts from Massachusetts Institute of Technology, Harvard University, and other organizations developed a machine learning model built to identify potential antibiotics based on their chemical structure. Their February 2020 article in Cell details the discovery of the halicin molecule’s antibiotic properties. Importantly, halicin was shown to be effective against resistant tuberculosis bacteria.
Halicin is a divergent molecule: its chemical structure and function is different from those of traditional ones (antibiotics in this case). Machine learning’s capacity to identify such divergent molecules presents a strong advantage over human methods, which often begin by finding candidates similar to successful antibiotics. While this method might seem logical, it could limit potential discoveries. Further, bacteria could be less likely to develop resistance to divergent antibiotics.
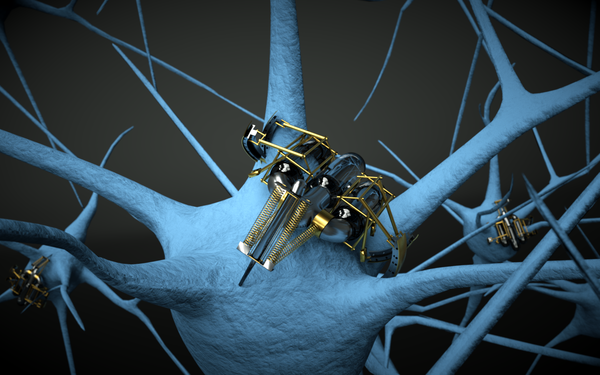
“Neurone” by Hugh Tomkins is licensed under CC BY-NC-ND 4.0
The machine learning model used to analyze halicin is called a deep neural network. It finds relationships within data using complex mathematics. Equipped with algorithms inspired by the human brain, a deep neural network can be trained with an initial data set—almost like a practice run—to then use the learned patterns to predict information about new data. Halicin’s antibiotic potential was realized after training a neural network to comb through a library of over 6,000 molecules. As one might imagine, machine learning can analyze that volume of data much more quickly than humans.
With such powerful algorithms, machine learning is beginning to meet the urgent need for treating resistant bacteria. As such, halicin is hopefully just one of many molecules that deep neural networks can identify as antibiotics. The outlook is promising. The same neural network that identified halicin analyzed another chemical library called ZINC15, this time with over 100 million entries. The algorithm identified another eight antibiotic candidates, two of which were shown to have broad-spectrum activity.
“Their approach highlights the power of computer-aided drug discovery. It would be impossible to physically test over 100m compounds for antibiotic activity,” Jacob Durrant of the University of Pittsburgh, a drug design researcher who was not part of the study, tells The Guardian.
In addition to killing tuberculosis-causing bacteria, halicin was shown to fight other resistant bacteria in mice models. For example, halicin strongly inhibited the growth of Acinetobacter baumannii bacteria. The World Health Organization states that this highly contagious bacteria, sometimes caught in hospitals, requires urgent new treatments.
Thanks to the efficiency of computers and cleverness of top researchers, the dangers of antibiotic resistant bacteria might become less likely.
Citation
Alex
"This title was very eye catching! That is so interesting that such a ..."
Alex
"This is really interesting! The fact that crops and plants are damaged is ..."
Alex
"Well done, this article is great and the information is very captivating! Ethics ..."
Alex
"I was intrigued throughout the whole article! This is such an interesting topic, ..."
Alex
"This is such an interesting article, and very relevant!! Great job at explaining ..."
Grandpa
"Honey You Did a good job I will forward to my eye doctor "
murphymv
"This article is fascinating because it delves into the details of the research ..."
murphymv
"I agree, adding the photo helped solidify the main finding. "
murphymv
"This is a fascinating finding. I hope this innovative approach to improving transplants ..."
Sherzilla
"This is a great article! I would really love to hear how exactly ..."
Sherzilla
"It's disappointment that these treatments were not very effective but hopefully other researchers ..."
Sherzilla
"I agree with your idea that we need to shift our focus to ..."
Sherzilla
"It's amazing to see how such an everyday household product such as ..."
Lauren Kageler
"I will be interested to see what the data looks like from the ..."
Lauren Kageler
"A very interesting article that emphasizes one of the many benefits that the ..."
maricha
"Great post! I had known about the plight of Little Browns, but I ..."
Sherzilla
"I assumed cancer patients were more at risk to the virus but I ..."
Sherzilla
"Great article! It sheds light on a topic that everyone is curious about. ..."
maricha
"This article is full of really important and relevant information! I really liked ..."
maricha
"Definitely a very newsworthy article! Nice job explaining the structure of the virus ..."
maricha
"It's interesting to think that humans aren't only species dealing with the global ..."
murphymv
"This is very interesting and well explained. I am not too familiar with ..."
Lauren Kageler
"Great article! This post is sure to be a useful resource for any ..."
Lauren Kageler
"Definitely seems like an odd pairing at first, but any step forward in ..."
murphymv
"What an interesting article! As you say, height and dementia seem unrelated at ..."
murphymv
"Great article! I learned several new methods of wildlife tracking. This seems like ..."
murphymv
"Very interesting topic! You explained cascade testing and its importance very well. I ..."
Alex
"This article is really interesting! What got me hooked right away was the ..."
Sabrina
"I found this article super interesting! It’s crazy how everyday products can cause ..."
Erin Heeschen
"I love the layout of this article; it's very eyecatching! The advancements of prosthetics ..."
murphymv
"Awesome article! I like the personality in the writing. Flash Graphene not only ..."
murphymv
"Very interesting work! I don't know a whole lot about genetics, but this ..."
Cami Meckley
"I think the idea of using virtual reality technology to better help prepare ..."
Erin Heeschen
"I wonder if there's a connection between tourist season and wildfires in the ..."
Ralph berezan
"Not bad Good work "
Michelle
"This sounds like it would be a great tool for medical students! ..."